Avoiding Unpleasant Surprises at the Investment Table
A strategic approach to risk based wind development
Introduction
A common complaint levied against wind projects in the later development stages is that not enough has been done to shore up estimates of how much energy the plant will produce. A confident energy prediction is the result of years of thoughtful planning and due diligence, and the wind resource assessment program is one of the most crucial undertakings of the pre-construction phase. Since the production estimate is usually the most sensitive value driver in a project pro forma, one wonders why the wind resource assessment program is so often an afterthought. The primary reason for this is that the market has not fully appreciated wind resource assessment risk, mainly because the classic approach to modeling wind resource assessment uncertainty is far too simplistic given the complexities involved, such as, measurement error, spatial variation, and climate uncertainties. The results can lead to less trustworthy conclusions with no way to truly differentiate between the riskiness of several projects. However, with a sophisticated risk model in place, it is possible to tailor investment much more sensitively to risk. By using future project uncertainty as a barometer, this model allows stakeholders to make decisions today with the singular goal of balancing current constraints against a future risk profile preset to the comfort level of the project’s developer and financiers.
Energy risk framework
It is important to build an articulate model to help manage risk. The framework is actually a collection of models, each one based on physical realities which are tied together in a software platform. The uncertainties are allowed to interact with each other through global covariance models. The framework attempts to capture the uncertainty of every step along the way, allowing that uncertainty to propagate through the entire model to the final measure of wind power performance risk. Each uncertainty model is tied to validation studies or statistical theory, providing a precise rationale for the structure of the model. A key highlight of this framework is the ability to model future energy-production assessment uncertainty by comparing various scenarios and comparing the outcomes before making investment decisions about the project. For example, if the developer is choosing between measurement technology types, such as met towers, sound detection and ranging (SoDAR), or light detection and ranging (LiDAR), they simply need to test the appropriate models created for that technology.
Consider a typical choice made in a wind resource assessment program: determining how much additional meteorological equipment to install at a site. The common way a developer would approach this would be with rules of thumb and best practices. Such rules might include: each project must have a hub height measurement, in simple terrain each project should have at least three met towers per 100MW of installed capacity, and in complex terrain five met towers per 100MW. This type of guidance is reasonable, but with a sophisticated uncertainty model, one can do so much better.
In Application
As an example, a developer is managing a 150 MW project in rolling farmland. The project has a single met tower that has collected one year of data. The company needs to have the project ready for significant investment decisions in the second quarter of the following year. The developer’s investment risk appetite requires that the ten-year energy uncertainty of any project be less than 8.0%, in other words, actual energy produced is likely within 8.0% above or below the initial estimate. With just the one met tower, current uncertainty is 11.5%. With limited time and budget, it is difficult to know the best way to invest to reduce uncertainty to an acceptable level for financing. If no additional met tower is added, it is expected the uncertainty will drop to just 11.3% by the time the Q2 investment decisions arises. The model reveals small gains in uncertainty reduction come from additional data, but the lack of spatial coverage limits how much gain is possible. So, an uncertainty optimization program is conducted.
The developer examines the impact on calculating uncertainty by adding met towers to the program, allowing each met mast to collect a year of data – the time remaining until the final investment evaluation must be made. Each met tower’s location is optimized to find the locations that have the greatest impact on the total, final uncertainty. With this logic, hundreds of scenarios are modeled, testing each configuration of met towers. From this analysis, it is discovered that if two additional met towers are installed in the next two months, uncertainty will dip below 8.0%, satisfying internal criteria by the time the investment decision must be made. The analysis also shows that after a total of three additional met towers are installed, uncertainty reduction has reached the point of diminishing returns. In other words, additional met towers will yield slight gains, but not enough to justify their upfront capital costs.
Knowledge of how quickly the uncertainty-reduction process can be sped up by installing equipment and collecting data, enables stakeholders to understand how much they must invest at every stage of project development. The developer also has the option to accelerate the wind resource assessment program while minimizing expense.
Comparing technologies
This approach is also useful for examining questions about technology choices. For example, what is the difference between remote sensing technologies? What about the difference between installing an 80-metre met tower or a 100-metre tower? An energy risk framework can be used to develop an investment strategy that minimizes required investments while optimizing where investment capital is best spent.
Risk based investment strategy
A sufficiently sensitive risk model enables the process of risk based wind project investment. Beginning with the end in mind, each investor should define its own tolerance for risk. After observing numerous uncertainties achieved at the project-investment stage, a rating system may be used as a guide, shown in Table 1. Projects are most often financed at a rating of C or better, which is a reasonable target.
With an investment-risk profile, it is possible to begin the task of managing a portfolio of investments on a track that leads each project to the desired risk level by the time final investment commitments must be made. This usually means the signing of a power-purchase agreement or turbine-supply agreement. Along the way, each developer should have milestones, attached to uncertainty levels which correspond to development stages within the pipeline. Unfortunately, no company has unlimited resources, and impacts from current decisions will often only be felt 1 to 2 years into the future. Each investor is forced to balance future risks against current budgets. Relying solely on rules of thumb and past experiences, rather than a risk model which can handle these decisions, is not the most objective and cost effective approach. Also, without a strong scientific justification for the need for expenditure, the wind resource assessment program can suffer from the ever-tightening budget requirements each company faces.
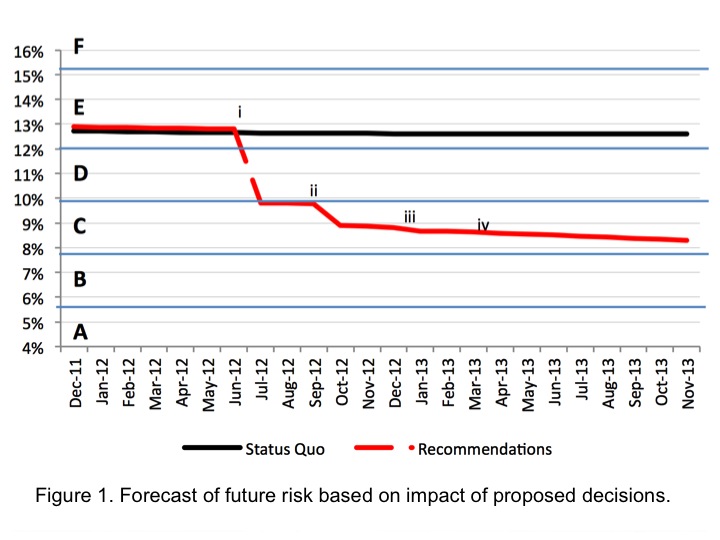
Implications
Understanding wind project risk is paramount to the ongoing success of this industry. Much underperformance in the industry can be linked to poor understanding and management of risk. Next generation uncertainty models, are required to properly navigate energy production risks. Any institution investing in this space should have a clear idea of its risk profile and equip itself with the tools to ensure that its portfolio adheres to a quantified profile. In wind project development, time is the resource most often squandered. Typically, there is just one chance to make the best decision. An optimized program is one that uses risk modeling as a regular part of its portfolio development strategy. When done properly, this helps reduce the chances of unpleasant surprises at the investment table.
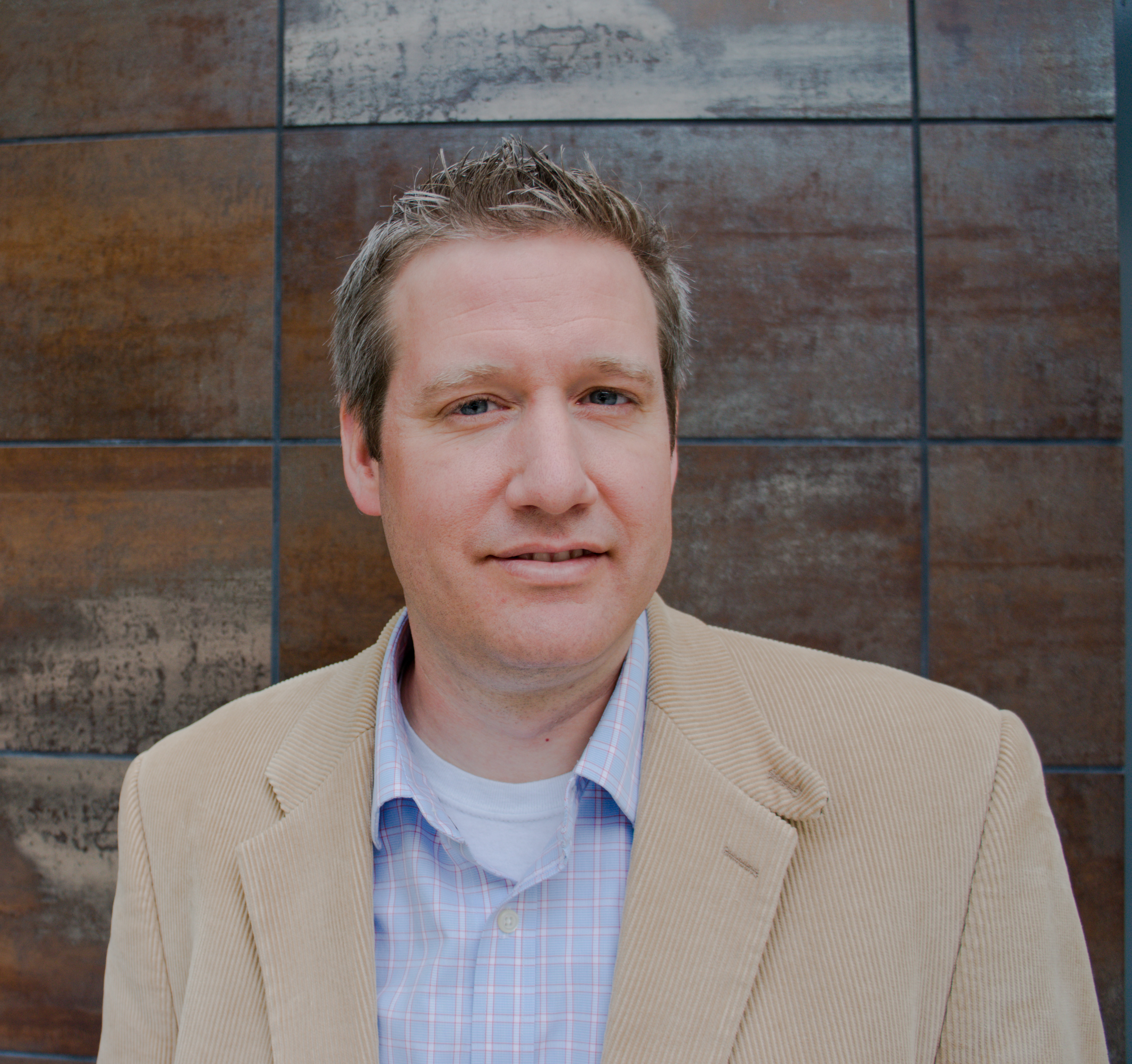
Mr. Hendrickson has extensive technical knowledge having personally managed energy assessment programs at EDP Renewables, involving more than 4GW of operating wind farms and a development pipeline of 20GW from 2003 to 2011. He received his electrical engineering degree from the University of Houston. Vaisala has developed a proprietary model called the Energy Risk Framework to measure future project uncertainty to help clients understand and mitigate wind power performance risk.
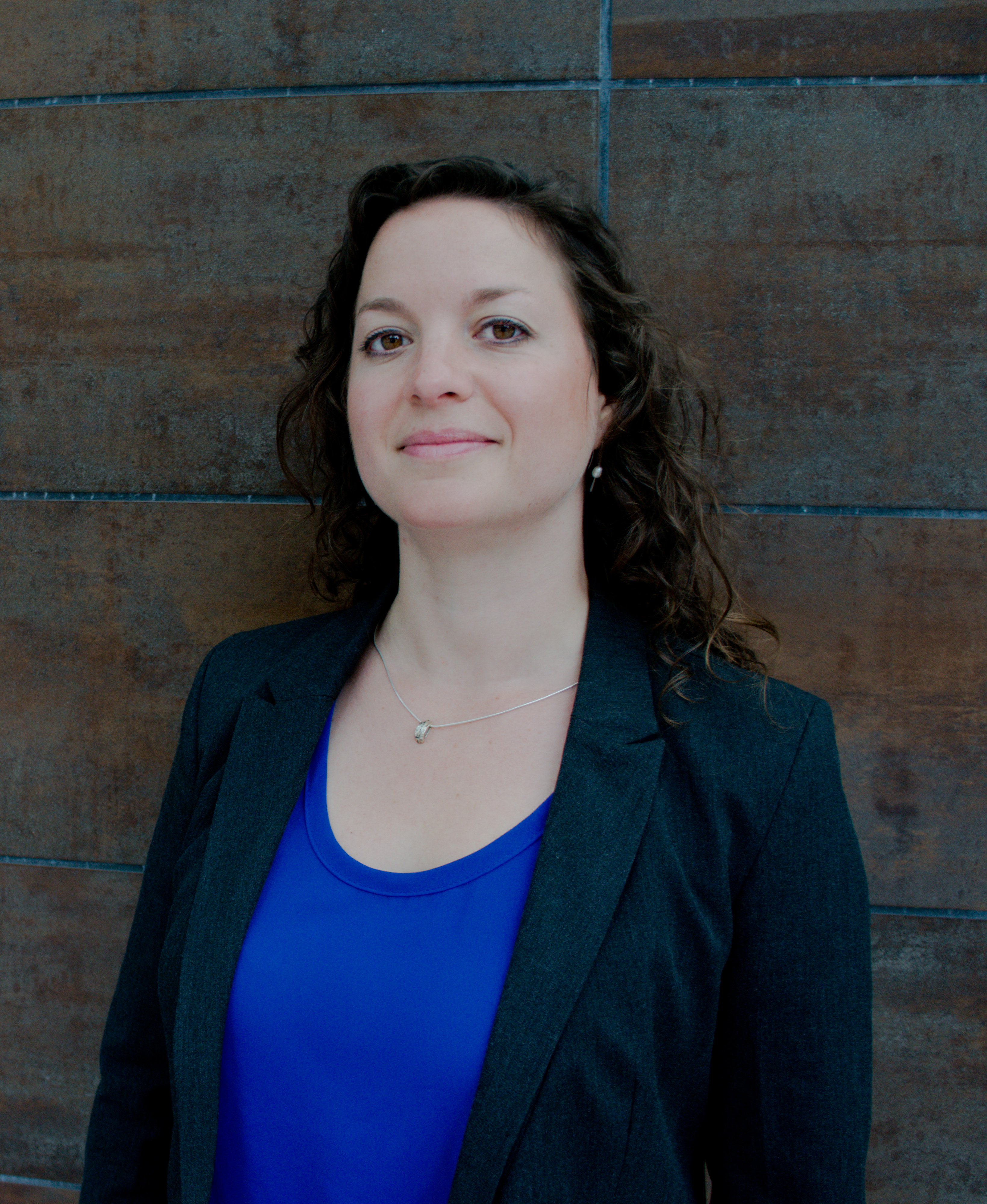
Vaisala, Inc. | www.vaisala.com
Volume: September/October 2015